by Nathan
The first post in this series gave a brief outline of Dr. Paul Sullins’ recent paper on homosexuality and clergy abuse, discussing an initial concern related to his use of data. The second post discussed correlation and the aggregation of data. This post will continue this discussion, outlining some basic issues of polynomial transformation. We hope to help equip Catholics to better evaluate data presented on this very controversial issue. We have linked within the article to definitions of various terms, and we highly recommend you reviewing these, as Catholics only stand to gain from an understanding of how statistics can be used and misused to support positions.
In order to support the hypothesis that abuse is related to homosexuality in the priesthood and homosexual subcultures in seminary, Sullins builds a multiple linear regression model to further quantify the relationships in Table 1. Although he does not take the simple step of examining the correlations between the variables on a disaggregated basis, testing the hypothesis via a model is a good next step. The model, or rather models, are summarized in the following table:

The table can be broken into a few areas of interest for now. Let’s start with the title (A): from it, we can gain some important background on the data used for this particular model. It announces that the data used is from the John Jay reports. In addition, from the “n=51” at the end we can infer that this model was fit on yearly, rather than five-year-bucketed data, as noted previously. (This makes it even more odd that the correlations in the yearly data was not reported in the paper, as we know now that Sullins examined the data in this format.)
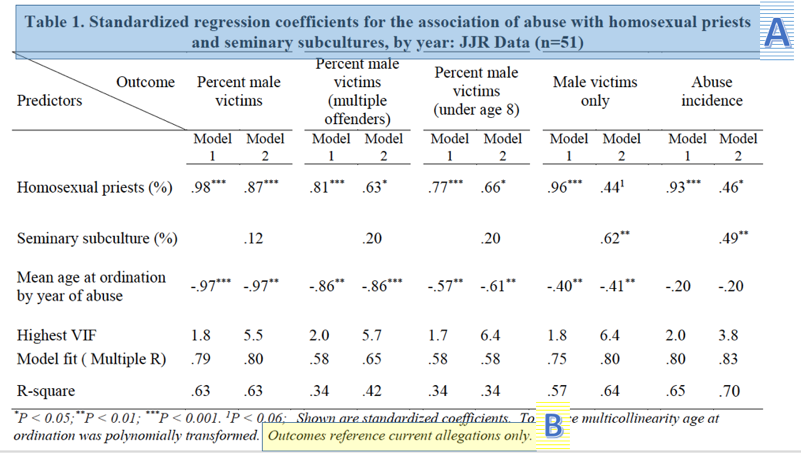
Finally, if we skip down to the last sentence of the footnote (B), we see that the data on which the model was fit was restricted to “current allegations.” Again, this is a definition Sullins uses elsewhere in the paper; it means allegations that were raised in the same year as the abuse. Sullins thus excludes the vast majority of allegations from his models and most-cited figures. Discerning readers should be concerned that this may be an arbitrary definition that threatens to introduce bias into the model.
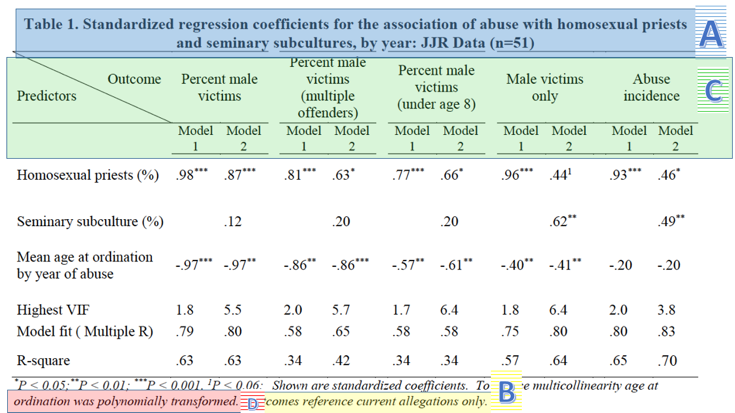
Moving down the table, the headers show the outcome variable in each model (C). For each outcome variable, two models were fit. “Model 1” includes the percent of homosexual priests. “Model 2” includes both that variable and the percent of priests reporting a homosexual subculture at their seminary. To both of these models Sullins added the mean age at ordination by year of abuse, which, skipping down again to the footnote, we find was “polynomially transformed” in order to “reduce multicollinearity” (D).
Multicollinearity is a technical pitfall of the class of models to which multiple linear regression belong. It occurs when two or more predictor variables are highly correlated with each other, and it causes the estimates of the model coefficients to be unreliable. This explains why Sullins would want to avoid it, but not why he included this variable in the model in the first place. It appears nowhere else in the paper and has nothing to do with the hypotheses he is testing with these models.
Did you not understand any of the above paragraph? Check out this video on multicollinearity:
Furthermore, Sullins does not explain the transformation applied to the variable, which is highly irregular. As standard practice, research papers typically explain both the data used and the model form in full. Unlike most professional papers, this paper omits all such explanations and expects the reviewer to blindly trust this transformation of the data.
In the next post, we will explain the standardized coefficients and summary statistics used by Sullins.
Posts in this series:
3. Polynomial Transformation
4. Coefficients
Comments